Mirrored Mastery - Harnessing Digital Twin Technology
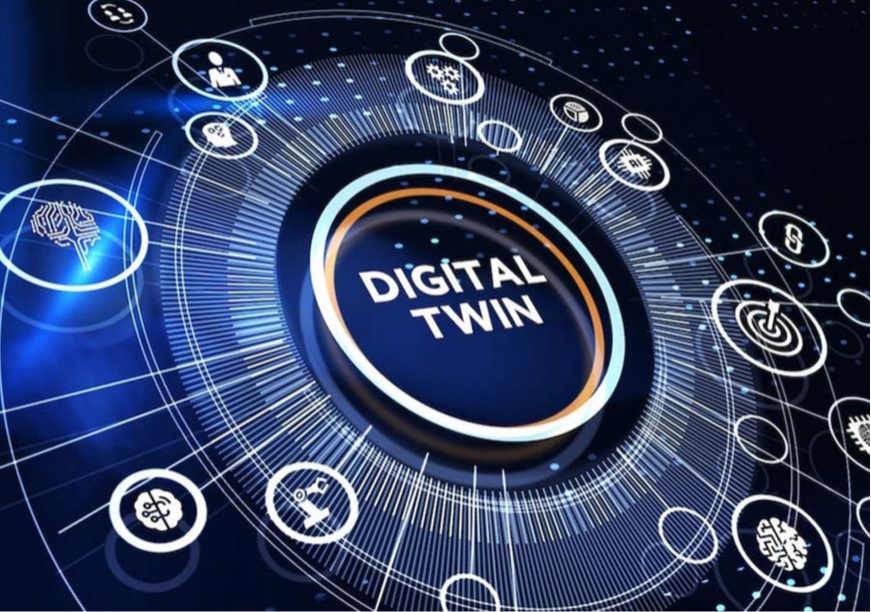
The Conceptual Convergence of Physical and Virtual
The concept of Digital Twin technology has revolutionized the way we approach the convergence of physical and virtual worlds. At its core, a Digital Twin is a virtual replica of a physical object, system, or process that mimics its characteristics, behaviors, and performance in real-time.
Definition of Digital Twin and its core principles
A Digital Twin is a dynamic, virtual representation of a physical entity that uses real-time data and other sources to simulate its behavior, performance, and lifespan. The core principles of Digital Twin technology include:
- Virtualization: Creating a virtual replica of a physical object or system
- Real-time data integration: Using sensors, IoT devices, and other data sources to update the virtual model in real-time
- Simulation and analysis: Using the virtual model to simulate scenarios, predict performance, and analyze behavior
- Continuous learning: Using data and insights to improve the virtual model and the physical object or system it represents
Historical background and evolution of the concept
The concept of Digital Twin has its roots in the early 2000s, when the term was first coined by Michael Grieves at the University of Michigan. Initially, it was used to describe the virtual modeling of complex systems in the aerospace industry. Over the years, the concept has evolved to encompass a broader range of applications, including manufacturing, healthcare, and urban planning.
Key characteristics of Digital Twin technology
Digital Twin technology has several key characteristics that make it a powerful tool for convergence of physical and virtual worlds:
- Real-time data integration and analytics
- Virtualization and simulation
- Continuous learning and improvement
- Interoperability and integration with other technologies
- Scalability and flexibility
Twinning Assets, Processes, and Systems
Digital twin technology has evolved to encompass a broad range of applications, transforming the way we approach asset management, process optimization, and system integration. In this section, we will delve into the various types of digital twins, their applications across industries, and the benefits they offer.
Types of Digital Twins
- Component Twins: These digital replicas represent individual components or parts of a larger system, enabling real-time monitoring and predictive maintenance.
- Asset Twins: Asset twins simulate the behavior and performance of physical assets, such as equipment or machinery, allowing for optimized operation and maintenance.
- System Twins: System twins model complex systems, including interactions and interdependencies, facilitating holistic analysis and optimization.
- Process Twins: Process twins replicate business processes, enabling simulation, analysis, and improvement of workflows and operational efficiency.
Applications across Industries
- Manufacturing: Digital twins optimize production processes, predict equipment failures, and improve product quality.
- Healthcare: Digital twins model patient behavior, simulate treatment outcomes, and optimize resource allocation.
- Urban Planning: Digital twins simulate city infrastructure, traffic flow, and energy consumption, enabling data-driven urban development.
Benefits of Digital Twinning
- Efficiency: Digital twins optimize resource allocation, reduce waste, and streamline processes.
- Predictability: Digital twins enable predictive maintenance, reducing downtime and improving overall system reliability.
- Innovation: Digital twins facilitate experimentation, testing, and validation of new ideas, accelerating innovation and growth.
The IoT and Data-Driven Dynamics
In the realm of Digital Twin Technology, the Internet of Things (IoT) plays a pivotal role in creating a dynamic, data-driven ecosystem. This section delves into the significance of IoT sensors, real-time data, data analytics, machine learning, and cyber-physical systems in harnessing the full potential of Digital Twinning.
Role of IoT sensors and real-time data in Digital Twinning
IoT sensors are the eyes and ears of Digital Twin Technology, generating vast amounts of real-time data that breathe life into digital replicas. These sensors monitor various parameters, such as temperature, pressure, and vibration, providing a constant stream of information that enables accurate simulations, predictions, and decision-making.
Data analytics and machine learning integration
The integration of data analytics and machine learning algorithms is crucial in extracting valuable insights from the IoT sensor data. By analyzing this data, organizations can identify patterns, detect anomalies, and predict potential outcomes, ultimately optimizing their operations and improving overall efficiency.
Cyber-physical systems and their significance
Cyber-physical systems (CPS) are the backbone of Digital Twin Technology, bridging the physical and digital worlds. CPS enables bidirectional communication between the physical entity and its digital twin, facilitating real-time monitoring, control, and optimization. This synergy between the physical and digital realms unlocks new possibilities for innovation, productivity, and growth.
Implementation and Integration Strategies
Implementing Digital Twin technology requires a well-structured approach to ensure seamless integration with existing systems and infrastructure. Here's a comprehensive guide to help you navigate the process:
Step-by-Step Approach to Implementing Digital Twin Technology
1. Define the scope and objectives: Clearly outline the goals and requirements of your Digital Twin project, including the assets to be replicated and the desired outcomes.
2. Assess existing infrastructure and systems: Evaluate your current technology stack, data management processes, and infrastructure to determine the necessary integrations and upgrades.
3. Select the right tools and platforms: Choose suitable Digital Twin software and tools that align with your objectives and infrastructure.
4. Develop a data management strategy: Establish a robust data management plan to handle the vast amounts of data generated by Digital Twins.
5. Build and test the Digital Twin model: Create a virtual replica of your asset or system, and conduct thorough testing to ensure accuracy and reliability.
6. Deploy and monitor the Digital Twin: Launch the Digital Twin and continuously monitor its performance, making adjustments as needed.
Integrating Digital Twins with Existing Systems and Infrastructure
1. API integrations: Utilize APIs to connect Digital Twins with existing systems, enabling seamless data exchange and synchronization.
2. Data harmonization: Ensure compatibility and consistency of data formats and structures across systems.
3. Cloud and on-premise integrations: Integrate Digital Twins with cloud-based and on-premise infrastructure, facilitating scalability and flexibility.
Change Management and Organizational Considerations
1. Develop a change management plan: Address potential organizational and cultural impacts of Digital Twin adoption.
2. Training and upskilling: Provide comprehensive training for employees to ensure they can effectively work with Digital Twins.
3. Establish clear roles and responsibilities: Define ownership and accountability for Digital Twin management and maintenance.
Navigating Challenges and Future Directions
Common Challenges and Limitations of Digital Twin Adoption
While Digital Twin technology holds immense potential, its adoption is not without challenges. Some common hurdles include:
- High upfront costs and investment requirements
- Complexity in integration with existing systems and infrastructure
- Data management and analytics challenges
- Security and privacy concerns
- Limited expertise and talent pool
- Organizational change management and cultural adoption
Future Trends and Advancements in Digital Twin Technology
As Digital Twin technology continues to evolve, we can expect:
- Increased adoption of cloud-based and hybrid architectures
- Advancements in AI, machine learning, and predictive analytics
- Integration with emerging technologies like AR, VR, and IoT
- Improved data management and analytics capabilities
- Enhanced security and privacy measures
- Expanded applications across industries and use cases
Best Practices for Ensuring Scalability and Sustainability
To ensure successful implementation and long-term benefits, consider:
- Start small and scale gradually
- Develop a clear business case and ROI framework
- Establish a cross-functional team and governance structure
- Invest in employee training and upskilling
- Monitor and evaluate performance regularly
- Prioritize data quality, security, and privacy
Comments ()