AMD vs Nvidia for AI - Performance, Power, and Price
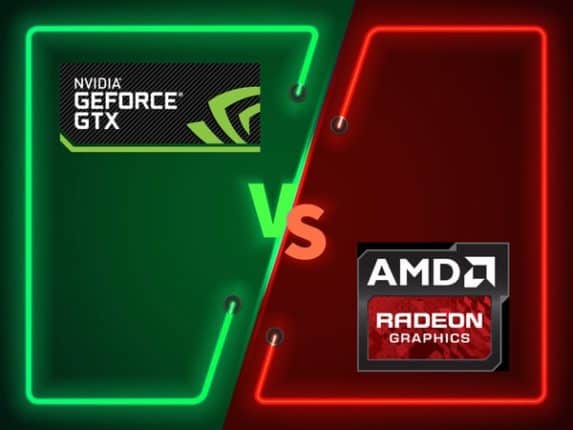
AMD's Strengths and Weaknesses
When it comes to AI, AMD has both strengths and weaknesses that impact its performance, power, and price competitiveness. Here's a detailed breakdown:
Strengths:
Competitive Position in AI Inference and Server CPUs
AMD has made significant strides in the AI inference and server CPU market, offering competitive solutions that rival Nvidia's dominance. Their EPYC series of server CPUs provides a strong foundation for AI workloads, offering high core counts, memory bandwidth, and PCIe lanes.
Better Power Efficiency and Cost-Effectiveness
AMD's GPUs, particularly the Instinct MI series, offer better power efficiency and are more cost-effective than Nvidia's equivalent offerings. This makes them an attractive choice for data centers and cloud providers looking to optimize their energy consumption and reduce costs.
Weaknesses:
Developing Software Ecosystem
While AMD has made significant progress in developing its software ecosystem, it still lags behind Nvidia's mature and extensive ecosystem. AMD's ROCm (Radeon Open Compute) platform, while improving, still requires further development to match Nvidia's CUDA platform in terms of tools, libraries, and community support.
GPU Performance Comparison
Nvidia's Performance Advantage
Nvidia's GPUs have consistently demonstrated higher compute performance in AI-related tasks, thanks to their advanced architecture and large number of CUDA cores. This advantage is particularly evident in applications that rely heavily on matrix multiplication and convolutional neural networks (CNNs). Nvidia's high-end GPUs, such as the A100 and GH200, offer unparalleled performance, making them the preferred choice for many AI researchers and professionals.
AMD's Radeon Instinct Series
AMD's Radeon Instinct series is specifically designed for AI applications, offering a range of GPUs that cater to different needs and budgets. The Instinct series boasts impressive performance, particularly in applications that utilize OpenCL and ROCm (Radeon Open Compute). AMD's GPUs also offer competitive power consumption and pricing, making them an attractive option for those looking for a more affordable AI solution.
Memory and Bandwidth Comparison
When it comes to memory and bandwidth, AMD's MI300X chip stands out with its impressive 192GB of memory, surpassing Nvidia's GH200, which offers 141GB. This significant difference in memory capacity can be crucial for large-scale AI applications that require massive amounts of data processing. Additionally, AMD's MI300X chip boasts a higher memory bandwidth, ensuring faster data transfer and processing.
Software Support and Ecosystem
Nvidia's extensive software support and ecosystem give it a significant edge in the AI landscape. Nvidia's CUDA platform, TensorRT, and cuDNN libraries provide a comprehensive toolkit for AI development, making it easier for researchers and professionals to optimize and deploy their models. In contrast, AMD's ROCm platform, while improving, still lags behind Nvidia's offerings in terms of maturity and adoption.
Power Consumption and Pricing
AMD's GPUs generally offer competitive power consumption and pricing compared to Nvidia's offerings. The Radeon Instinct series, in particular, provides a range of options for those looking to balance performance and power efficiency. Nvidia's high-end GPUs, while incredibly powerful, often come with a significant power consumption penalty, which may be a concern for data centers and organizations with limited power budgets.
Investment and Stock Comparison
Nvidia: A Stronger Choice for AI Investors
Nvidia is widely regarded as a more attractive option for investors seeking to capitalize on the burgeoning AI market. Its dominance in the field of deep learning and AI computing has led to significant revenue growth, making it an appealing choice for those looking to invest in AI technology.
AMD's Stock: Potential for Growth, but Higher Volatility
While AMD's stock has shown potential for growth, it is generally considered more volatile than Nvidia's. This increased volatility can be attributed to AMD's broader focus on various markets, including CPUs and GPUs for gaming and professional applications, in addition to AI. As such, investors should exercise caution and thoroughly assess their risk tolerance before investing in AMD's stock.
Conclusion
After examining the performance, power consumption, and pricing of AMD and Nvidia GPUs for AI applications, it's clear that both companies have their strengths and weaknesses. In conclusion, the choice between AMD and Nvidia GPUs ultimately depends on specific AI requirements and budget constraints.
AMD's Advantages in AI
AMD's GPUs offer several advantages in AI workloads, including:
- Competitive performance in training and inference tasks with its RDNA and CDNA architectures
- Lower power consumption, resulting in reduced energy costs and increased datacenter efficiency
- More affordable pricing options, making high-performance AI computing more accessible
- Open-source ROCm platform, promoting developer collaboration and customization
Nvidia's Dominance in AI
Nvidia's GPUs maintain a strong lead in AI due to:
- Unmatched performance in complex AI workloads, such as deep learning and natural language processing
- Extensive support for popular AI frameworks, including TensorFlow and PyTorch
- Advanced Tensor Core and CUDA technologies, accelerating matrix operations and parallel processing
- A vast ecosystem of developers, researchers, and partners, driving innovation and optimization
Choosing the Right GPU for AI
When selecting a GPU for AI applications, consider the following factors:
- Specific AI workload requirements (e.g., computer vision, NLP, or recommender systems)
- Compute resources and memory needs (e.g., VRAM, tensor cores, or FP32/FP16 performance)
- Power consumption and cooling constraints
- Software compatibility and framework support
- Budget and total cost of ownership
By carefully evaluating these factors and weighing the strengths of AMD and Nvidia GPUs, developers, researchers, and organizations can make informed decisions to optimize their AI infrastructure and achieve breakthrough results.
Comments ()